はじめに
こちらの記事はZennで投稿した「Keras Tunerでハイパーパラメータチューニング」の再投稿になります。
kaggleでKerasによるニューラルネットワークモデルのハイパーパラメータチューニンがしたく、Keras Tunerを使ってみました。
今回は、Titanicコンペで実際に使ってみながら紹介していきます。
対象読者
- Kerasのモデルでハイパーパラメータチューニングしたい
- Keras Tunerを使ってみたい
ゴール
- Keras Tunerでハイパーパラメータチューニングができる
Keras Tunerとは
Kerasでハイパーパラメータチューニングができるライブラリです。 層やユニットの数、どの層を使うかなど、モデルを構築する上で様々なパラメータをチューニングすることができます。
Titanicコンペでやってみる
実際にTitanicコンペのデータを使ってKeras Tunerを使っていきます。 https://www.kaggle.com/c/titanic
データの前処理の詳しい説明については、割愛しています。
インストール
まずはKeras Tunerをインストールします。
1pip install keras-tuner
インポート
チューニングするRandomSearch
をインポートします。
1from kerastuner.tuners import RandomSearch
モデルの定義
Keras Tunerでパラメータチューニングできるようにモデルを定義していきます。
今回設定しているパラメータは以下の4つになります。
- 層の数 (2~20)
hp.Int('num_layers', 2, 20)
- ユニットの数 (32~512の32ごと値)
hp.Int('units_' + str(i), min_value=32, max_value=512, step=32)
- BatchNormalizationかDropoutかどちらもか
hp.Choice('batchnorm_and_dropout', ['batch', 'dropout', 'both'])
- 学習率 (1e-2, 1e-3, 1e-4)
hp.Choice('learning_rate', [1e-2, 1e-3, 1e-4])
1def build_model(hp):
2 model = keras.Sequential()
3 for i in range(hp.Int('num_layers', 2, 20)):
4 model.add(layers.Dense(units=hp.Int('units_' + str(i),
5 min_value=32,
6 max_value=512,
7 step=32),
8 activation='relu'))
9 if hp.Choice('batchnorm_and_dropout', ['batch', 'dropout', 'both']) == 'batch':
10 model.add(layers.BatchNormalization())
11 elif hp.Choice('batchnorm_and_dropout', ['batch', 'dropout', 'both']) == 'dropout':
12 model.add(layers.Dropout(0.2))
13 else:
14 model.add(layers.BatchNormalization())
15 model.add(layers.Dropout(0.2))
16 model.add(layers.Dense(1, activation="sigmoid"))
17 model.compile(
18 optimizer=keras.optimizers.Adam(
19 hp.Choice('learning_rate', [1e-2, 1e-3, 1e-4])),
20 loss=keras.losses.BinaryCrossentropy(from_logits=True),
21 metrics=[keras.metrics.BinaryAccuracy(name='acc')])
22 return model
今回はhp.Int
とhp.Choice
を使いましたが、他にも種類があります。
https://keras-team.github.io/keras-tuner/documentation/hyperparameters/
チューニング
定義したモデルを使ってインスタンス化します。
今回はRandomSearch
を利用してチューニングをします。
1tuner = RandomSearch(
2 build_model,
3 objective='val_acc',
4 max_trials=100,
5 overwrite=True)
https://keras-team.github.io/keras-tuner/documentation/tuners/
チューニング実行中のコールバックを定義できます。EarlyStopping
を利用するとチューニングの実行を短縮できます。
1callbacks=[keras.callbacks.EarlyStopping(monitor='val_acc', mode='max', patience=3)]
チューニング実行していきます。
与えられた学習データ(X, y)
を、学習データと検証データに分けて利用します。
1X_train, X_val, y_train, y_val = train_test_split(X, y, test_size=0.3)
2tuner.search(X_train, y_train, validation_data=(X_val, y_val), callbacks=callbacks, epochs=100)
チューニングしたパラメータを使う
最も良かったパラメータでモデルを構築して、学習します。
1best_hp = tuner.get_best_hyperparameters()[0]
2model = build_model(best_hp)
3model.fit(X, y, epochs=1000)
今回は以下のようなモデルになりました。
1Model: "sequential_1"
2_________________________________________________________________
3Layer (type) Output Shape Param #
4=================================================================
5dense_4 (Dense) (None, 256) 2560
6_________________________________________________________________
7batch_normalization_3 (Batch (None, 256) 1024
8_________________________________________________________________
9dense_5 (Dense) (None, 128) 32896
10_________________________________________________________________
11batch_normalization_4 (Batch (None, 128) 512
12_________________________________________________________________
13dense_6 (Dense) (None, 256) 33024
14_________________________________________________________________
15batch_normalization_5 (Batch (None, 256) 1024
16_________________________________________________________________
17dense_7 (Dense) (None, 256) 65792
18_________________________________________________________________
19batch_normalization_6 (Batch (None, 256) 1024
20_________________________________________________________________
21dense_8 (Dense) (None, 1) 257
22=================================================================
23Total params: 138,113
24Trainable params: 136,321
25Non-trainable params: 1,792
26_________________________________________________________________
学習したモデルでの予測を提出したらスコアは0.73684でした。
まとめ
- Keras Tunerでハイパーパラメータチューニングが簡単にできる
kaggleのKernelも作成したので、良かったら見てください。
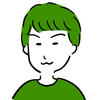
Titanic: Hyperparameter tuning with Keras Tuner
Explore and run machine learning code with Kaggle Notebooks | Using data from Titanic - Machine Learning from Disaster
参考
- Keras Tuner
- Keras Tuner の基礎 | TensorFlow Core
- keras-team/keras-tuner: Hyperparameter tuning for humans